All Things Data
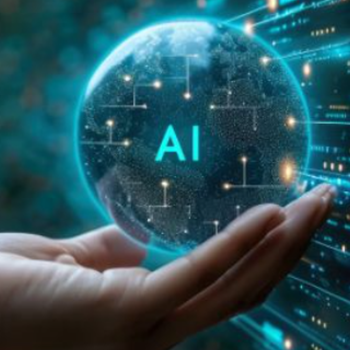
Key Takeaway:
"All Things Data" represents a broad, integral shift in data management strategies driven by the need for greater efficiency, strategic insight, and technological integration. The trend encompasses various facets of data management, from application-specific optimizations to advanced GenAI integrations, reflecting the evolving demands and opportunities within modern business environments. In 2024, this shift will not merely be about managing data but transforming it into a pivotal strategic asset. Furthermore, in a recent BCG survey, 68% of companies enquired plan to implement next-generation data architectures within the next three years, highlighting the broad-based commitment to evolving data strategies to meet contemporary and future demands better.
Trend Type: Technology
Sub-trends: Application performance as measure of data management, Management visibility into database operations, GenAI implications on data management, Organizations adopt autonomic data man-agement, Customer Data Revolution, GenAI need for data accessibility and mobility, DevOps de-mand for automated data control, Next-Gen Data Architecture, GenAI Enterprise Data, Synthetic data bonanza, Machine Customers
Sub-Trend Sources
Application performance as measure of data management: BMC Blogs IT Trends
Management visibility into database operations: BMC Blogs IT Trends
GenAI implications on data management: BMC Blogs IT Trends, Kyndryl, Kantar's Media Trends, Finance & Development
Organizations adopt autonomic data management: BMC Blogs IT Trends
Customer Data Revolution: eTail Connect European Playbook, Thoughtworks Tech Radar
GenAI need for data accessibility and mobility: BMC Blogs IT Trends
DevOps demand for automated data control: BMC Blogs IT Trends
Next-Gen Data Architecture: BCG The Next Wave
GenAI Enterprise Data: Delloite TMT Predictions
Synthetic data bonanza: CBInsights Emerging Tech Trends
Machine Customers: Gartner Strategic Trends
What to Read Next
Wise Spending
Euromonitor’s data reveals a pressing concern for 74% of consumers in 2023: the escalating cost of everyday items. This issue has prompted a significant shift in consumer behavior, with a[...]
Eco Tech
The “Eco Tech” trend reflects the growing integration of technology with environmental sustainability efforts. Among the forefront technologies is carbon capture. Industries such as cement and steel are increasingly adopting[...]
Quantum Computing
Latest Advances Although Quantum Computing was already present in last year’s Digital Trends, in 2024, the race to develop viable quantum systems is intensifying, with tech giants like IBM, Google,[...]
What to Read Next
Wise Spending
Euromonitor’s data reveals a pressing concern for 74% of consumers in 2023: the escalating cost of everyday items. This issue has prompted a significant shift[...]
Eco Tech
The “Eco Tech” trend reflects the growing integration of technology with environmental sustainability efforts. Among the forefront technologies is carbon capture. Industries such as cement[...]
Quantum Computing
Latest Advances Although Quantum Computing was already present in last year’s Digital Trends, in 2024, the race to develop viable quantum systems is intensifying, with[...]